A tree’s roots beneath the surface communicate with each other and share nutrients, powering life on Earth. Well, that's a lot like data, but instead of powering our natural world, it's the bedrock of many of our technological systems. Looking back to the earliest forms of record keeping, data has been a key pillar in how we leverage knowledge to make valuable decisions that help guide our future.
Exploration and science have allowed us to learn about previously unreachable places vital to understanding our existence — oceans, space, remote forests — similarly, research-driven breakthroughs in technology have allowed us to harness and decipher the internet's vast expanse of data to generate new outcomes and opportunities. But this has not been easy. The mammoth task of getting great insights has been time-intensive, costly, and complicated. Across the many industries that depend on data analytics, the process of acquiring good data is lengthy, expensive, and requires multiple resources for labeling and organization. But imagine if it didn’t have to be?
As we wrote in our introduction article, we owe the improvement of generative AI's ability to detect and classify unstructured data — and ultimately generate new content or insights — largely to more and better data, research-powered breakthroughs, and more available compute. Thanks to deep learning and generative AI’s ability to decipher both structured and unstructured data sets, we can use most of the data available to us.
Shingai Manjengwa, head of AI Education at ChainML, defines data science and analytics as, “the process of ethically acquiring, engineering, analyzing, visualizing and ultimately creating value from data.” Users and businesses can do just that, create value from data and the role of ‘analytics’ is more important than ever.
What does this look like in action?
Enter ‘Council’ by ChainML, an open-source platform for rapidly developing customized generative AI applications using collaborating ‘agents’. Among many use cases, Council can help power self-service data analytics as a tool for our world’s businesses, creators, investors, developers, and more. Through Council, we can visualize data through charts and analytical dashboards tailor-made for client products. We can truly ‘speak to the data’ and now the data talks back.
A Conversation With Data
Here's an example of how generative AI-powered, self-service data analytics can inform decisions using our Conversational AI Engine:
Suppose we’re a game developer working on a new launch. We want to know what kind of games, features, trends, skins, and sounds are fuelling trends in player activity and leading to adoption. How do we find that information? Sure, we could spend countless hours playing games, talking to gamers, studying reports, and scrolling forums, and while that would be fun, it may not be the best use of our time.
Apps like ChatGPT can propose a strategy for the launch or provide some research findings, but they can't implement or execute that strategy. AutoGPT might generate a plan for how to proceed but it may get stuck in a loop without producing anything of value. A more strategic approach would be to think about how a team of experts — including a Research Agent and SQL Engineer — could support a human user/requester — develop an AI Model to generate analytical insights that can inform better strategies and even product design. Imagine a conversation among multiple entities called ‘agents’. These agents perform services.
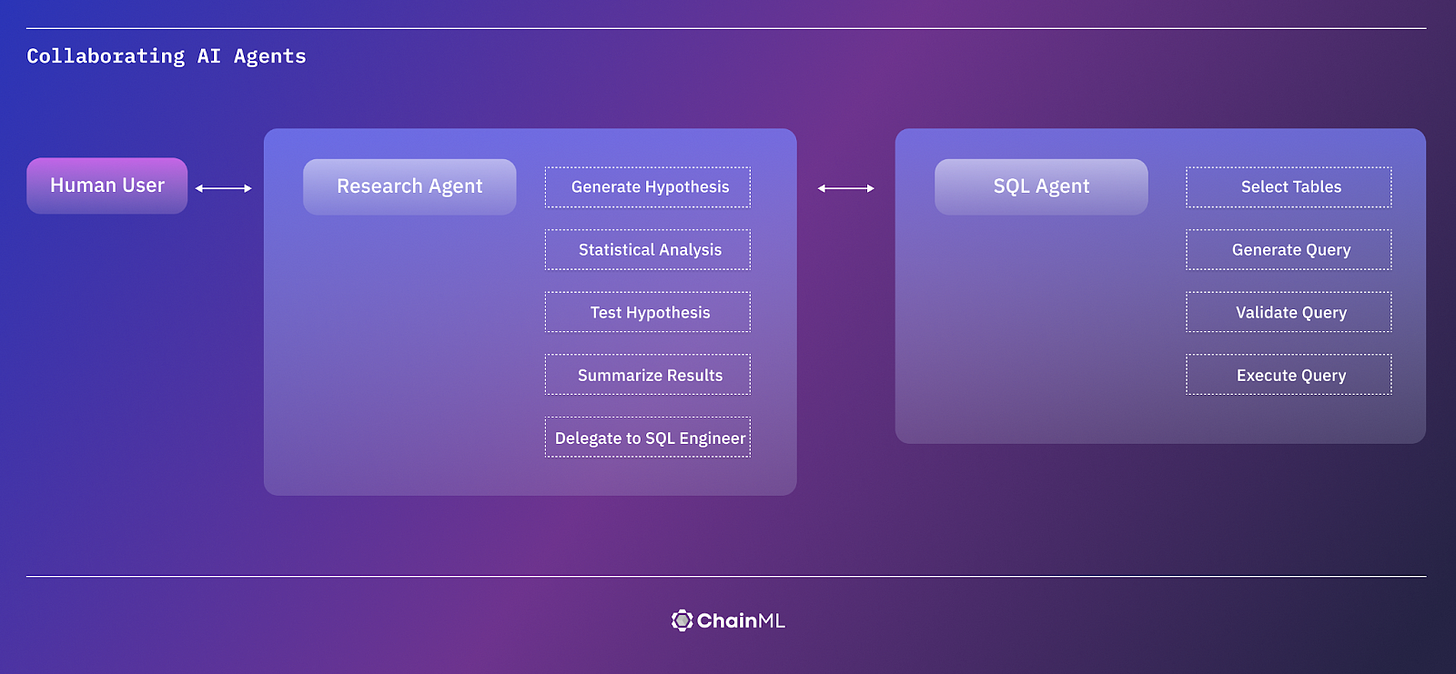
Recall we’re a game developer working on a new launch and we want to know drivers of player activity that lead to adoption. The image shows how Council can help us address this problem by simulating the roles described above, the Research Manager and the SQL Engineer. Here we have a Research Agent generating the hypothesis and conducting analyses and the SQL Agent that writes the queries to extract the right data for the Research Agent to use. The user receives the insights they need as though they had a team of real experts working with them.
Self-Service Analytics: In Action
Self-Service analytics is a generative use case we're especially excited about — and building for — at ChainML. We have co-built, 'Houston,' a generative AI-powered dApp for our client and partner, Space and Time. Space and Time is a "decentralized data warehouse" that enables text-to-SQL queries for building analytical data dashboards, applications, and much more. The feedback so far has been great:
“We are excited to be working with ChainML's Council to enable Houston's industry-leading AI functionality and power the ability to generate dashboards and build data pipelines with natural language prompts.” — Scott Dykstra, Space and Time Co-Founder & CTO

A look into some of the potential use cases of self-service analytics made possible by generative AI, Council, and chatbots like Houston.
Houston can index vast sets of data — like support docs or financial market data, as demonstrated in this Loom tutorial — to provide clear and concise outputs that translate technical infrastructure and data into actionable insights.
Houston is a powerful example of how to think of AI as a team with different skills working together to solve a problem. In our game design example, two agents were able to query, retrieve, and analyze data, and process it for visualization in the front end in a fast and efficient way.
The end result was a predictive and actionable analysis to advance the user’s objective.
The Future of Businesses in AI, Powered by Agents
But this story began with data, the bedrock of technology systems. Research-powered breakthroughs have created more opportunities for developers to harness data to build and deploy applications that allow consumers and businesses to improve productivity, optimize products, and educate themselves in new and exciting ways informed by powerful analytics.
In time, more users will gain the ability to leverage data through the growing adoption of next-generation AI chatbots and the large language models which help power them. They will need key roles (data scientists, application developers, ops, etc.) to build models and applications that enable impactful use cases. One way to achieve this is through reliable and scalable AI agents that can be deployed with confidence.
Interested in building self-service analytics into your application? Contact us on our website.
Stay connected to ChainML on Twitter.
Join the community on Discord, explore and toss us a (⭐) on GitHub.