2023, the Year of AI?
If the first few weeks of this year are anything to go by, 2023 is the year of AI. The hype is hard to ignore - and it’s difficult not to be excited. Technologists and mainstream users alike celebrate the recent breakthroughs in generative AI, exemplified by OpenAI’s successful launch of the impressive ChatGPT and the ever improving quality of image generative models like Stable Diffusion and Midjourney. Venture capital is deployed to fund a rapidly growing number of AI startups, while influencers meme about the shift of developer interest from Web3 to AI.
Web3 and AI
In Web2, the state-of-the-art in AI research and applications has long been driven by the largest companies, above all technology leaders such as Google (and DeepMind), Microsoft, Amazon or Meta. Much of the success and industry leadership of these companies comes from a successful AI strategy, their widespread use of advanced AI in technologies and services.
But so far, Web3 has been lagging behind in the AI arms race. While many projects across DeFi, gaming, social, content creation and infrastructure (among many other application areas and verticals) could massively benefit from incorporating AI capabilities into products that expand functionality to gain or extend an edge over the competition, the Web3 AI revolution has yet to take place.
Web3 AI Use Cases
The benefits of AI for Web3 projects and protocols are obvious. AI use cases exist across all major Web3 verticals addressing challenges in application areas like finance, security, user experience, content creation and governance.
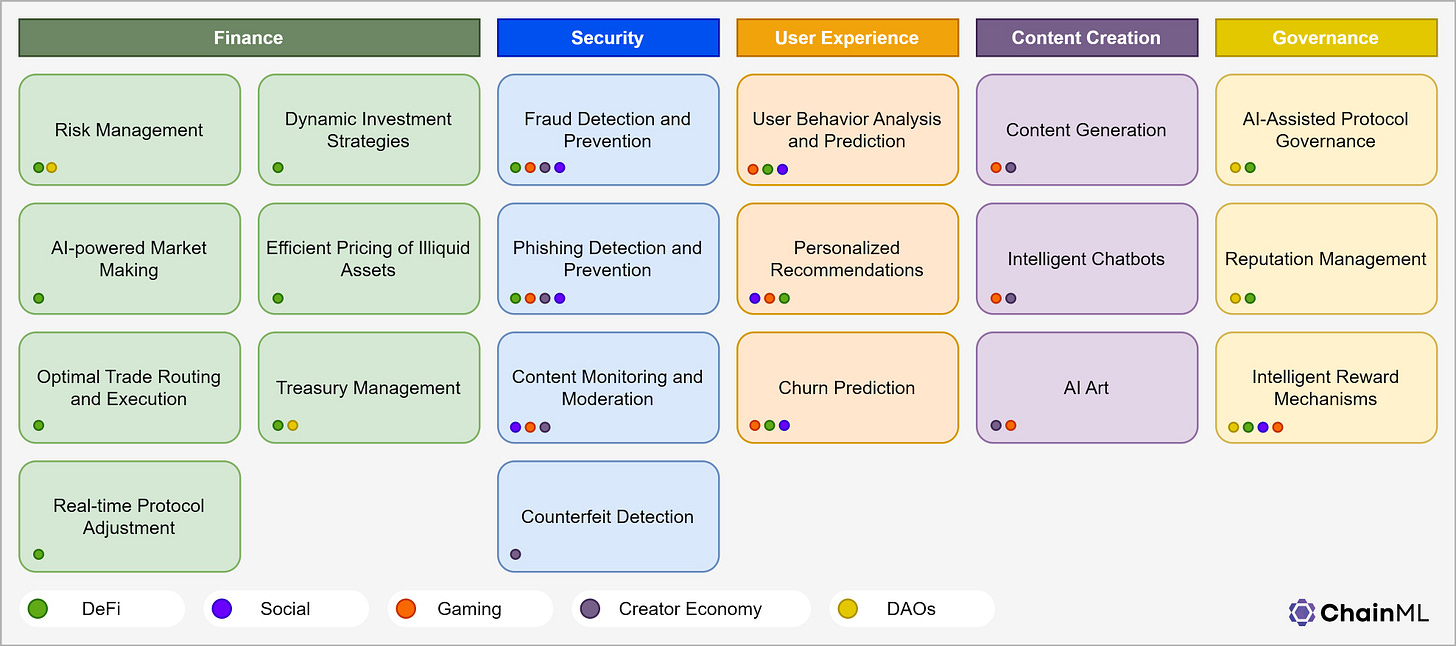
Finance
AI and ML models allow DeFi protocols to assess and manage risk associated with protocol activity and transactions, such as credit risks, liquidity risks, or market risks. This allows protocols and their users to better manage and mitigate risks while capturing more economic benefits and making more informed and confident decisions. DeFi applications can also leverage AI/ML to forecast the price and volatility of assets, such as cryptocurrencies, stablecoins, and other digital assets. This enables efficient pricing of illiquid assets, efficient trade execution under favorable market conditions and improved treasury management including adapting investment strategies (such as managing liquidity pool concentration) based on changing conditions. AI models support the automation and optimization of market making and liquidity provision processes, allowing platforms to offer more efficient and effective liquidity services. AI can be applied to improve the speed and accuracy of trade execution whether on a DeX, a DeX aggregator, for a DAO’s treasury, a liquidity pool strategy, or a wallet. For example, ML algorithms can be used to identify the best market makers or liquidity providers to execute a given order, or to optimize the routing of orders to maximize liquidity and minimize slippage.
Let’s look at how AI algorithms can be used to identify and set fixed collateral ratios for liquidation protection in lending protocols. Recent attacks, such as the Mango exploit and CRV attacks on Aave, have shown how attackers can manipulate asset prices to leave a decentralized autonomous organization (DAO) with significant losses from insufficient collateral. AI-powered real-time models for liquidation protection offer a new approach to protecting against these types of attacks and improve the stability of lending protocols. These models use real-time data and predictive models to continuously monitor the value of collateral and make on-the-fly adjustments to loan terms and conditions in order to minimize the risk of liquidation and ensure timely liquidation when necessary, to avoid draining protocol treasuries to cover losses.
A possible way to implement the use of AI for real-time risk management in lending protocols is the following:

AI models require access to live and historical market data such as trade history across Automated Market Makers, including contextual information like liquidity, token ownership and float, and Centralized Exchange market data. This is important because the value of collateral is constantly changing, and real-time data is needed to accurately monitor and assess the risk of liquidation. Datasets feed into a first AI model which predicts the risk of a given position falling below the loan amount (state-of-the-art deep learning forecasting models have proven to do well at this task in our internal tests). A second model predicts how long it will take to unwind a position. Long unwind processes can have a significant impact on the stability of the lending ecosystem and therefore increase risk. The results of both models are then used to produce updated risk parameters.
Security
AI services can help detect and prevent fraud and increase the security and trustworthiness of Web3 protocols and transactions. By analyzing large amounts of data, these algorithms are able to identify patterns and anomalies that may indicate fraudulent or malicious activity, and alert users or platform operators to take appropriate action to prevent fraud and protect users. AI can be applied to monitor and moderate content and help secure the NFT ecosystem by detecting counterfeit or copyright infringing NFT assets.
User Experience
AI algorithms have a range of applications in Web3 user analytics, including user behavior analysis, behavior prediction and personalized recommendations. This allows protocols, marketplaces, games and social networks to identify patterns and trends within user behavior and provide personalized content and ranked recommendations that help improve the user experience and maximize user engagement and retention (therefore minimizing churn). In the context of Web3 social networks, content recommender systems are applied to source, rank and share content meaningful to each individual. This can include posts that are of predicted interest to a user, or connections to other members of the social network a user is likely to interact with. Decentralized social networks can enable users to run their own / preferred third-party algorithms for content filtering and recommendation, therefore providing “algorithmic choice”.
Content Creation
Recent advances in generative AI models (including GPT-powered text generative models and text-to-image models like Stable Diffusion and Midjourney) provide a tremendous opportunity to automate the creation of content, including automated creation of NFT assets, with significant implications to the entire Web3 creator economy. We previously wrote about this in the context of Web3 gaming.
Let’s look at the example of asset generation in on-chain gaming: Text-to-image generative AI models that are fine-tuned to automate the generation of image game content from text descriptions have found their way into the gaming world. In a Web3 context, smart contracts can be used to govern the creation of game assets such as character portraits, weapons, vehicles, items and artifacts, with user ownership tracked as NFTs and, optionally, allowing users to participate in the generation process by providing their own descriptions. Likewise, state-of-the-art language models can be called to add context (such as item power statistics, characteristics or lore) within allowed limits to the generated assets. The following graphic exemplifies this workflow.

Governance
Predictive models can help improve and automate governance and decision-making processes of Web3 communities and DAOs. This includes the application of advanced models to analyze and summarize data and feedback from users and stakeholders in order to identify patterns and trends that may inform and improve the decisions of protocol governance bodies. AI enables automation of reputation management and rewards based on how individuals are contributing to a community, rewarding those who are deeply and purposefully engaged (instead of giving out benefits to users who are farming for rewards without providing any value to a community).
Stay Tuned
This concludes part one of this series where we have looked at the benefits of AI for Web3. Stay tuned as we are going to shed light on how AI is able to benefit from Web3 technology and predict what 2023 has in store for the intersection of AI and Web3 in a follow up blog post.
About ChainML
ChainML (chainml.net) is a startup founded by experienced technology executives and machine learning experts that has raised $4M led by IOSG Ventures and supported by Hashkey Capital, LongHash Ventures, SNZ, strategic partners, and Silicon Valley executives. ChainML has graduated from AllianceDao’s accelerator program and is among the first 10 projects participating in Chainlink BUILD.
ChainML is building a tamper-proof protocol for decentralized computation to unlock artificial intelligence and machine learning and other complex data-rich computation for Web3, extending the power of blockchains. ChainML simplifies using AI/ML in smart contracts, dApps and wallets with easy integration, censorship resistance and scalability while enabling third party extensions for user choice of AI/ML algorithms.